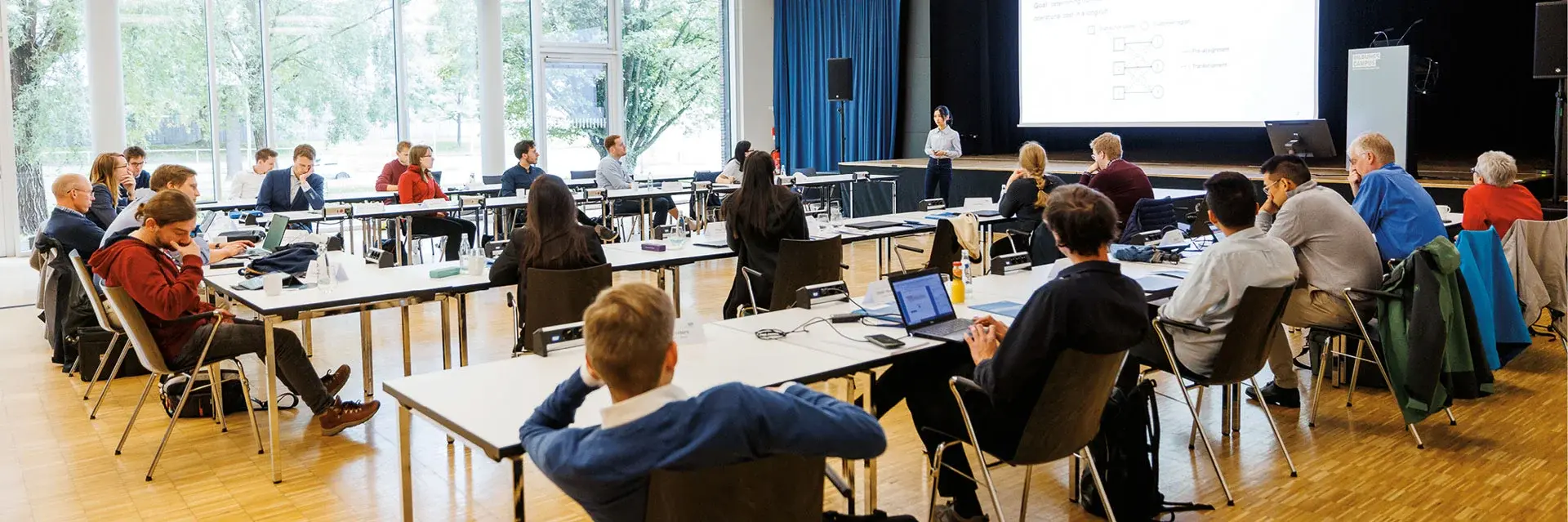
When AI Responds to Reward
Deep reinforcement learning is one of the most exciting research topics in the field of artificial intelligence. The technology could offer great benefits for companies in the future.
Modern household appliances make our everyday lives much easier. And when something is wrong, they even communicate with us. Cryptic error codes usually provide an initial indication of where the technical problem might lie. In some cases, a search query on Google helps, and the error can be fixed by hand. But there are also those where customer service has to come in to get to the bottom of the cause.
Let’s take a look at the repair service provider’s specialist in charge. When planning her workday, she asks herself two questions: what spare parts do I pack? And which route do I take to process as many orders as possible? The challenge: both factors are mutually dependent. Because of the error codes or the large variance in possible causes, there is a risk that the predicted number of spare parts will not be sufficient. If this is the case, an unscheduled visit to the workshop has to be undertaken, or another on-site appointment has to be scheduled. As a result, not only is the patience of everyone involved strained – the profitability of the company also suffers.
As of today, there is no solution to this problem – and certainly no efficient algorithm. Instead, the responsible employees fall back on their professional experience. But humans cannot, of course, take all variables into account in their planning. So the question arises: How can such decision-making processes be optimized in the future?
Deep Reinforcement Learning Holds Great Potential
The answer to this question is artificial intelligence. All over the world, science and industry are pinning great hopes on what is known as deep reinforcement learning (DRL) – a combination of deep learning and reinforcement learning. This AI method requires less expert knowledge than the classical programming of problem-specific solutions. Only the problem to be solved is defined in advance. Based on historical data sets, the AI simulates various scenarios in an interactive learning environment. For the execution of previously defined tasks, it receives immediate feedback in the form of a “reward” or “punishment.” Through this trial-and-error function, the AI independently learns which actions lead to the maximum reward and thus to the best possible task performance.
So much for the theory. In industrial practice, however, deep reinforcement learning has hardly been used so far. The reasons for this are complex: the AI method only works if companies provide a correspondingly broad database. This is often not available. In addition, DRL is extremely computationally intensive, and implementation is associated with a high level of effort. So it needs a high-performance IT infrastructure and competent employees. And last but not least: it is not uncommon for companies to have significant reservations about DRL. There is simply a lack of understanding of how the AI method culminates in a functioning strategy. So how can these issues be resolved to unlock the potential of DRL technology in the near future?
Workshop Provides Ideas, Insights, and Feedback
This question was part of a workshop that brought interested parties to TUM Campus Heilbronn last fall. The thematic focus of the three-day event was the application of deep reinforcement learning to dynamic decision-making problems in inventory management, transportation, manufacturing, and healthcare.
Led by a TUM trio consisting of Prof. Gudrun P. Kiesmüller and Prof. Jingui Xie from the TUM Campus Heilbronn and Prof. Stefan Minner from the TUM campus in Munich, about 30 high-ranking international experts discussed the current state of research and how the method can be used in operations management in the future. Several doctoral students from the TUM School of Management, TUM School of Computation, Information and Technology, and other international universities also had the opportunity to present their work and subsequently exchange ideas with the experienced researchers. An enriching experience, as Yihua Wang, TUM Ph.D. student from Munich, confirms: “I received valuable feedback and thank the organizational team for this fantastic event.”
"The exchange was great. But perhaps even more valuable was meeting so many new people who bring exciting new ideas to the field."
- Prof. Willem van Jaarsveld, Chair of Stochastic Optimization and Machine Learning at Eindhoven University of Technology
At the end of the workshop, it was clear to all participants that deep reinforcement learning opens up completely new possibilities for companies. In the future, it will be possible to optimize or automate processes that were previously considered too complex for software, in a variety of application areas. New and promising ideas have been developed, especially with regard to problems with large action areas. However, in order to exploit these potentials, the prerequisites must be created, and there are still some challenges to be overcome. Collaborations between science and industry are needed, as are good expertise and structured data provided by companies. Only in this way can DRL be implemented and further developed in practice.
Slider: When AI responds to reward
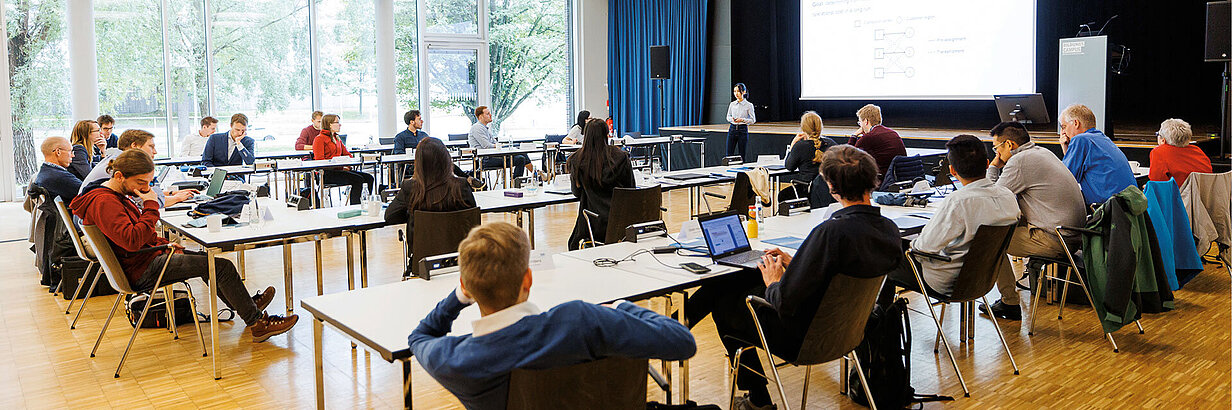